Shelly Sinclair, shsinclair@davidson.edu
This web page was produced as an assignment for an undergraduate course at Davidson College.
Click here to visit our homepage.
A genomic technique known as single-cell factor analysis network (scFAN) can predict binding patterns for proteins known as transcription factors in any single cell.
Understanding gene regulation and expression is essential in the field of genomics and plays a significant role in human health and disease. The dynamics of gene regulation and specifically the possible mutations that may occur are crucial in understanding the development of certain genetic illnesses (Araya et al. 2014). Studying the genome is important because it is the complete set of genetic information present in a cell (Boyle et al. 2009). Further, the genetic elements involved in gene regulation includes a vast array of proteins, RNA and DNA, collectively known as chromatin (Boyle et al. 2009). One example of a genetic element involved in gene regulation is transcription factors (Fig.1). These are proteins that have been identified within the genomes and play an active role in gene expression by targeting specific genes that have a variety of functions, such as development and physiology (Niu et al. 2011). Transcription factors have specific binding sites within the genome that are critical to know in order to understand how an organism will develop and function (Niu et al. 2011).
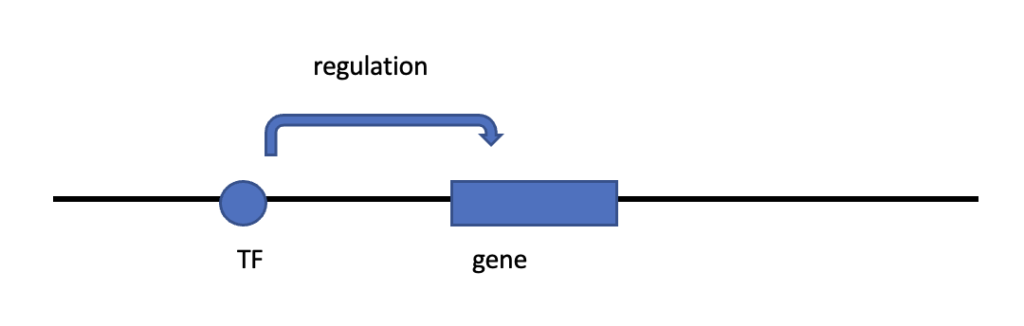
Fig.1 A depiction of the human genome. This is a simplified version of a genome with a transcription factor, gene and an arrow to illustrate the regulation process.
According to the article, “Predicting transcription factor binding in single cells through deep learning”, transcription factors bind to a region that is directly involved in aiding or inhibiting gene expression of a specified target gene. However, other research has identified binding regions of transcription factors in noncoding elements of a genome (Boyle et al. 2009). Essentially, the binding of a transcription factors is incredibly diverse and remains fairly unknown at a cellular level (Fu et al. 2020). These binding profiles are critical to understand because it effects which genes that transcription factor will target. Consequently, the genes that are targeted and expressed will affect how a cell differentiates (Fu et al. 2020). The identity of a cell characterizes cellular function which influences an organism’s development and growth (Niu et al. 2011). Therefore, different genomic techniques need to be used to develop the transcription factor binding profiles in the human genome. Previously identified techniques in other studies (Araya et al. 2014) have focused on obtaining binding patterns within a population of cells. While this information is certainly useful, it is still not targeted to identify the transcription factor binding profiles in individual cells to uncover the information of cellular identity (Fu et al. 2020). Therefore, the research question is as follows: How can transcription factor binding profiles be predicted and validated at a single-cell level?
Determining the binding profiles of transcription factors will aid in uncovering more on regulatory mechanism for genes and predicting cell differentiation and cellular identities (Fu et al. 2020). The authors of this primary research article used the single-cell factor analysis network to predict those transcription factor binding spots in individual cells. In order to obtain the single cell data, the researchers first obtained the binding data for a population of cells using different chromatin accessibility assays. Using a combination of DNA information along with the data from the chromatin accessibility assay, the researchers developed a metric to quantify the results. The metric to analyze this data, known as the transcription factor activity score, aided in characterizing each cell’s identity. scFAN provided an effective tool in this study that enabled researchers to predict different transcription factors binding patterns. In order to validate those predictions, different models were used to confirm those binding profiles and were compared to recurring patterns in DNA. Once confirmed, researchers could correlate the predicted binding profiles to the different cell types based on the activity score (Fig. 2).
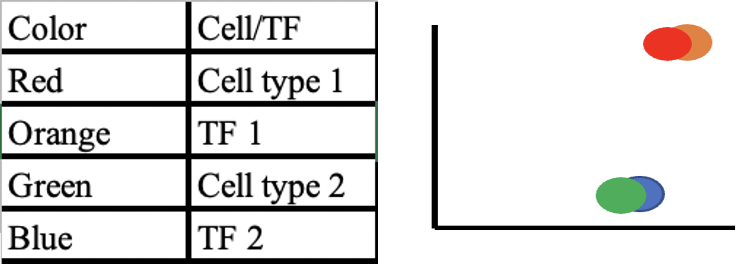
Fig. 2 Transcription factor activity scores correlates to cell type. This is a simplified graph of scFAN analyses that showed how the binding of a transcription factor was connected to a specific cell type.
The findings of this paper concluded that scFAN addresses the problems of chromatin assays that analyze cell populations by analyzing single-cells. Overall, scFAN was successful in predicting binding patterns of transcription factors that would elucidate information on cell differentiation and cell identities (Fu et al. 2020). The research further concludes that scFAN is a promising tool that can better enable understanding of cellular dynamics in humans. However, the research on this technique is far from over and more improvement is required. The datasets used in this study did not cover all the transcription factors that exist in humans so the transcription factor activity scores are incomplete. Experimental projects focused on validation of transcription factor activity is still needed because the datasets lack full coverage. Further research can examine the functional importance of different binding profiles in the human genome. Information taken from studies using scFAN could even play a role in how genetic diseases are addressed in medicinal research. Despite the necessity of future research on the subject, the work highlighted here shows that scFAN will prompt greater discovery and comprehension of gene regulatory mechanisms.
© Copyright 2021 Department of Biology, Davidson College, Davidson, NC 28036